A knowledge graph is a data structure that maps the relationships between sets of data in a network. A knowledge graph is made up of nodes and edges, where each node can belong to one or more subgraphs.
Nodes are typically nouns or entities (people, places, things) that have some meaning in the world. Edges are relationships between nodes, such as “lives in” or “works at.” The purpose of a knowledge graph is to help us understand patterns, relationships, and similarities between things. This allows us to discover insights into our world that we may not have been able to see before. To help users find relevant content, search engines such as Google often use knowledge graphs. Another example may be if you ask Siri a question about the weather, she might respond with something like “It’s going to be sunny.” This is helpful information for you because it helps you plan accordingly, but it’s also helpful for her because it allows her to find out more about you which expands her knowledge graph with helps her better understand you.This allows us to understand how information moves around systems or organisations, allowing you to see where it came from and how it got there.
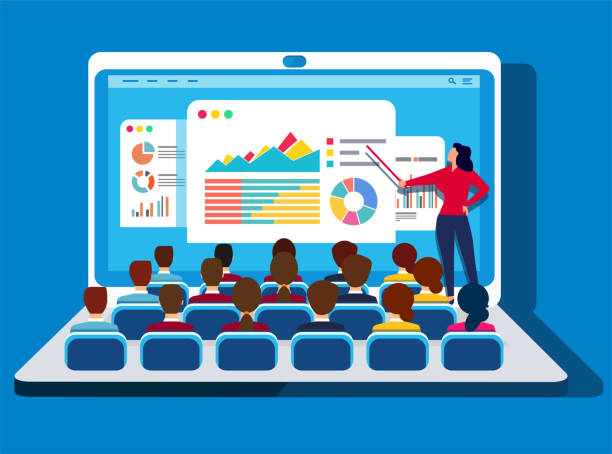
Benefits Of Using Knowledge graphs
The most important part about using knowledge graphs is that it makes it possible to see how certain pieces of data relate to others, which is important when working with data as it may have not been organised in that way before.
Knowledge graphs have many benefits that make them useful for organisations,here are a few :
– They allow you to find patterns in their data that would otherwise be difficult or impossible to find without using a knowledge graph model.For example that X goes to a specific coffee shop every monday morning.
– Knowledge graphs have many benefits, but one of the most important is that they allow organisations to see where they stand against competitors or other groups. By creating a knowledge graph, you’re able to get an in-depth look at how your organisation compares to others in terms of research and development, revenue, and more.
-Knowledge graphs can improve your business’s search capabilities, so you can find the information you need in a fraction of the time it would take if you had to manually search through your database of information
-Knowledge graphs allow you to visualise data in ways that are useful for both humans and machines, which ultimately increases efficiency in the workplace.
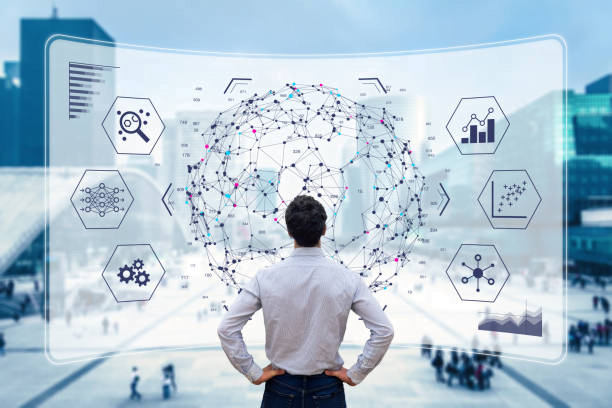
A Brief Description : The Interaction Between Knowledge Graphs And Machine Learning.
Knowledge graphs are a relatively new concept in the world of Artificial Intelligence, and they’re quickly becoming popular as machine learning becomes ever more intelligent.
Machine learning is a type of AI that uses computer programs to learn without being explicitly programmed. This means that the machine will be able to adapt and improve over time, as it learns from its experiences and observation of the world around it(Similar to you and i). Machine learning is also used in many other fields, including robotics and self-driving cars. Knowledge graphs are one way machine learning can be applied to data in order to help make sense of it.
Knowledge graphs allow machines to understand and interact with data. Machine learning algorithms can then use this information to formulate predictions based on prior experiences, such as knowing that people who own a particular car are likely to be younger than average or having access to a particular piece of information before making a decision about buying something online.