4 Types Of Dirty Product Data
‘Dirty product data’ refers to incomplete or inaccurate product information. Dirty product data can affect your business in many ways, including:
-Loss of revenue due to inaccurate inventory counts
-Inability to fulfil customer requests for products that are out of stock
-Unnecessary waste of resources due to mislabeled products
In this article we are going to discuss the four common types of dirty data.
#Incomplete Data
Incomplete product data occurs when a company does not have all of the necessary information about a product. As a result customers may be left with inadequate information about a product and this can lead them to using another business. Below are a few ways incomplete data may affect your business :
– Decreased brand loyalty
– It could result in errors when entering orders or processing payments.
– If you are trying to build awareness around your brand or product and use incomplete product data, it could give people misleading information about your products or services.
– Increased time spent informing customers on product information as they don’t have access to it.
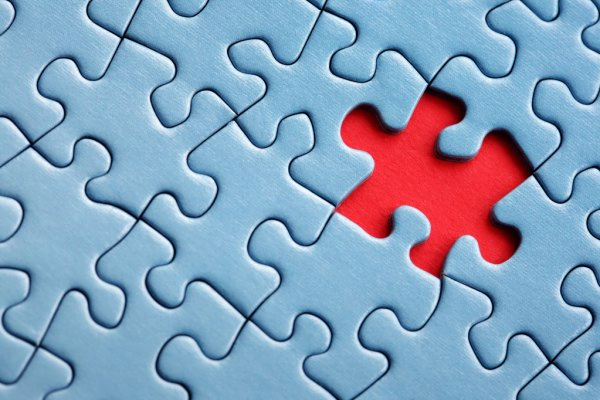
#Inaccurate Data
Inaccurate product data is any information that is incorrect or misleading. This can be caused by human or software errors and is a costly issue that businesses face. An example of inaccurate data is if a product had to be delivered to the wrong address resulting in timely delays for customers. In order to prevent inaccurate data,data needs to be collected properly,completely and accurately to prevent potential issues.
Below are some common causes of inaccurate data:
– Data is not being constantly reviewed
– Lack of data about a product or customer when filing takes place
– Lack of skills from employees on data management and software
– Out of date systems within a business
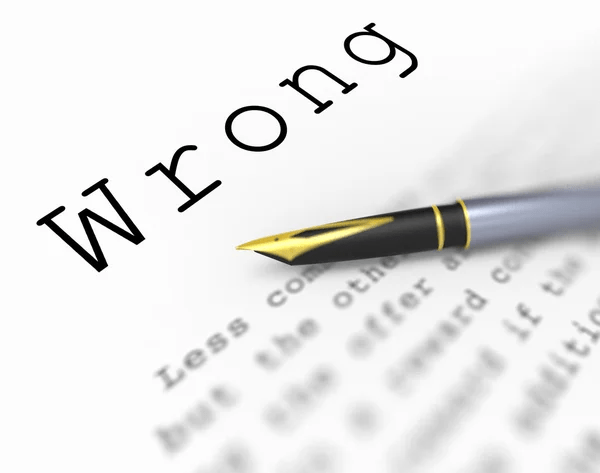
#Inconsistent Data
Inconsistent data is when the data you’re delivering to your business operations isn’t entirely accurate or consistent. This can have a variety of impacts on your business, both in terms of how you’re engaging with customers and how you’re conducting business activities.
The term inconsistent refers to data that does not match up with the way it was intended for, or the way you want it to be. For example, if a company is selling products online and has two different prices for the same items, they may have inconsistent data.
You also lose credibility if your customers don’t trust what they’re buying from you, so it’s important that you make sure all sales transactions are handled with utmost care and accuracy. This means making sure that orders are filled accurately and delivered on time (or as soon as possible).
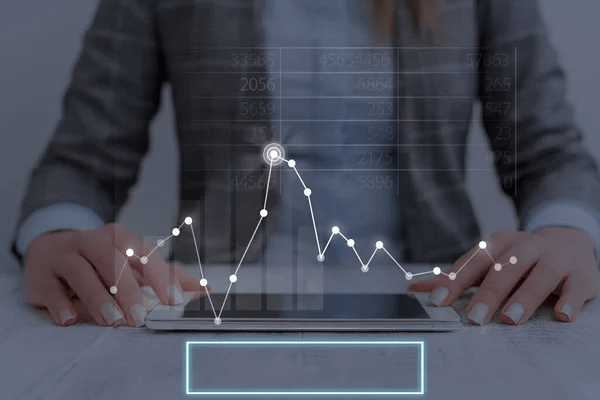
#Duplicated Data
Duplicate data is when you have more than one record for a particular field in your database. For example, if an employee’s name and ID number are entered into two different systems but they’re the same person, we’d say that this person has duplicate data.This can happen if someone inputs an incorrect value for the field, or if you accidentally use the same value twice.Duplicate data can be a problem if it’s not cleaned up properly.
Duplicate data can cause several problems for your business, including:
– Lost sales opportunities because customers are double-booked by two separate businesses.
– High-risk financial losses if your company relies on credit card payments and you accidentally issue payment twice to the same customer.
– Increased management costs if you need to hire additional staff members to manage duplicate records in your system.
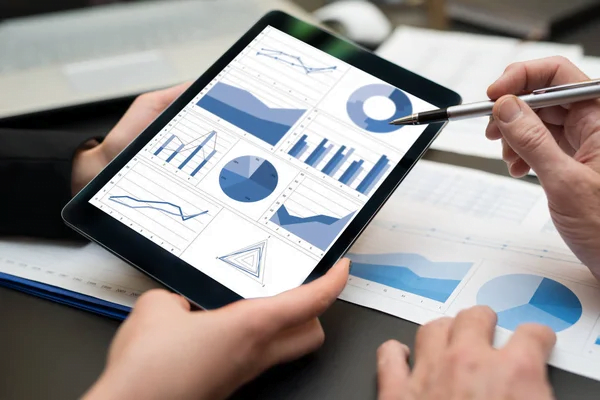
Conclusion
While dirty product data can be a costly and time consuming problem, getting rid of it doesn’t have to be. There are a number of ways to deal with dirty product data, but the most important thing is to start by identifying exactly what it is that you’re dealing with. Visit our website to find out how we can help you clean your dirty data
#dirtydata #dirtyproduct #dirtyproducts #dirtyproductdata #dirtyproductdataanalysis #dirtyproductdataexamples #dirtyproductdataset #dirtyproductdatasets #dirtyproductdatasetanalysis #dirtyproductdatasetexamples #dirtyproductdatasetmining #dirtyproductdatamining