How to Clean Your Dirty Product Data
We live in a digital age and as a result data has become the backbone of our everyday lives. In this article, we’ll discuss how to identify dirty data in your business and how to clean it to improve your internal and external operations.
Why Is Clean Product Data Important
The importance of clean product data lies in the fact that it helps you connect with your customers by showing the most relevant and user friendly data, but it also helps you find new customers. Having clean data helps you increase your business reach and this increases traffic to your website, as well as helping you reduce the time you spend manually entering information into systems. Clean product data can also allow you to focus on other tasks that require your attention.This is because resolving dirty product data can be a tedious task. Increasing profitability in your business is very important and having clean product data allows you to make informed decisions about inventory management and pricing which can help you achieve this . Furthermore, clean product data can make marketing your products easier, which leads to more traffic and sales.
Clean product data is a key factor in selling products online. When your product data is clean and accurate, you can:
-Improve your marketing ROI by offering customers the most relevant products and services
-Increase customer retention by ensuring they receive the products they ordered in a timely manner
-Reduce costs related to returns and refunds
How Do You Identify Dirty Product Data
Dirty Product Data is an issue that can plague a business if not dealt with properly, but it can be fixed.Dirty data can cause problems for your organisation because it’s not accurate, which means you might not be able to trust it. For example, if you want to analyse sales data by region, then dirty product data could mean that the information doesn’t match up. If you’re trying to track how many customers bought a certain product in each region, you’ll have a problem if there are inconsistencies between regions because the data isn’t clean or consistent. Here are some ways you can identify dirty product data:
Look at your products’ descriptions. Are they filled with spelling errors, or do they contain content that doesn’t make sense? If so, then this might be dirty product data.
Look at how many times your products have been searched for. If there are a lot of searches for one particular item and none for another, then the item that has had no searches may have bad data associated with it.
Look at your inventory count and compare it with what you sell each day. If there is a big difference between these numbers, then there may be some dirty product data going on in your store’s inventory system.
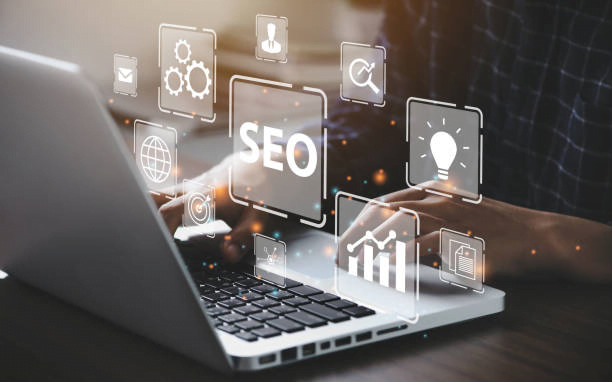
Ways You Can Clean Dirty Product Data
We know that cleaning dirty product data can be a tedious task:
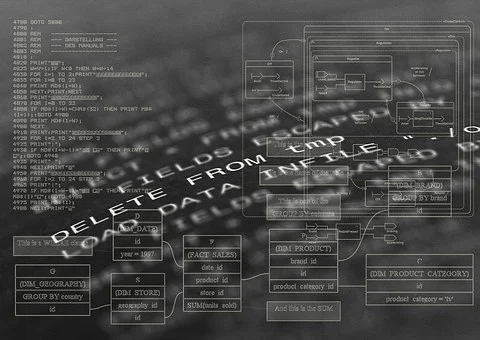
Here are some different ways you can clean your data:
1. Delete duplicates: If you have duplicates, delete them first. This will help reduce the size of your dataset and make it easier to work with.
2. Filter out bad rows: Look for rows that are missing data or have incorrect values. These should be filtered out or removed before doing any analysis.
3. Check for missing values: Look at all variables in your dataset to see if there are any missing values. If so, fill in those values with zeros or another appropriate value. It’s important that these missing values be filled in before doing any analysis because otherwise you won’t have complete data on which to base your conclusions!
4. Check for outliers: Outliers are observations that are very different from other observations within a dataset and may need to be removed before doing any analysis. They can skew results and make it difficult to interpret findings from a study because they don’t represent typical behaviour.